Understanding Deep Learning vs. Machine Learning
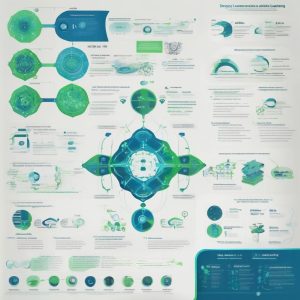
Understanding Deep Learning vs. Machine Learning.
Meta Description: Explore the complexities of deep learning and machine learning, two vital components of modern AI technology. Discover their differences, use cases, and how they shape the future of computing.
Introduction
In the fast-evolving world of artificial intelligence (AI), deep learning and machine learning are often discussed, but not always clearly understood. This article aims to clarify these concepts, explaining their differences, applications, and impacts on technology and business.
What is Machine Learning?
Machine learning (ML) is a subset of AI that focuses on building systems that can learn from, and make decisions based on, data. It utilizes algorithms to identify patterns, enabling machines to improve their performance over time without being explicitly programmed.
Key Characteristics of Machine Learning:
Supervised Learning: Utilizes labeled data to train algorithms.
Unsupervised Learning: Deals with unlabeled data, discovering hidden patterns.
Reinforcement Learning: Involves agents that learn optimal actions through rewards and punishments.
What is Deep Learning?
Deep learning is an advanced subset of machine learning that mimics the workings of the human brain in processing data and creating patterns for use in decision making. It employs neural networks with three or more layers—often referred to as deep neural networks.
READ ALSO: Top 5 Ways to Monetize Your Blog in 2024.
Key Characteristics of Deep Learning:
Complex Architectures: Uses artificial neural networks.
Feature Automation: Reduces the need for manual feature extraction.
Large Data Handling: Excels in processing large sets of data.
Deep Learning vs. Machine Learning: Key Differences
Data Requirements: Deep learning requires large datasets, whereas machine learning can manage with smaller sets.
Hardware Dependencies: Deep learning is computationally intensive, often requiring GPUs.
Accuracy and Performance: Deep learning generally delivers higher accuracy but at the cost of increased complexity.
Training Time: Deep learning models usually take longer to train.
Application Scope: Machine learning is used in simpler tasks, whereas deep learning addresses more complex issues, such as image and speech recognition.
Applications of Machine Learning
Fraud Detection: Machine learning algorithms can identify fraudulent activities by analyzing patterns.
Recommendation Systems: Services like Netflix and Amazon use ML models to suggest relevant content to users.
Customer Segmentation: Helps businesses segment their market for targeted advertising.
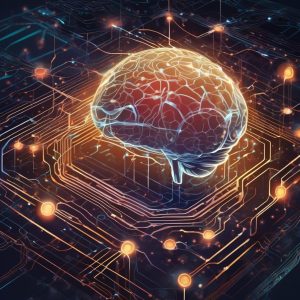
Applications of Deep Learning
Natural Language Processing (NLP): Enhances understanding and response to human language.
Autonomous Vehicles: Used in self-driving car technology to interpret sensory data.
Medical Diagnosis: Assists in diagnosing diseases in radiology and pathology.
Recent Trends and Future Directions
AI Integration Across Industries: More sectors are adopting AI to automate processes.
Ethical AI Development: Growing importance of ethical considerations in AI deployment.
Continued Research and Innovation: Ongoing research to improve efficiency and effectiveness.
Conclusion
The journey of understanding deep learning vs. machine learning reveals a spectrum of capabilities and potential. While both technologies stem from AI, their differences highlight varied functionalities and applications. Embracing these technologies paves the way for innovation and efficiency in multiple domains.